Customer Segmentation Using AI: Smarter Insights, Better Results
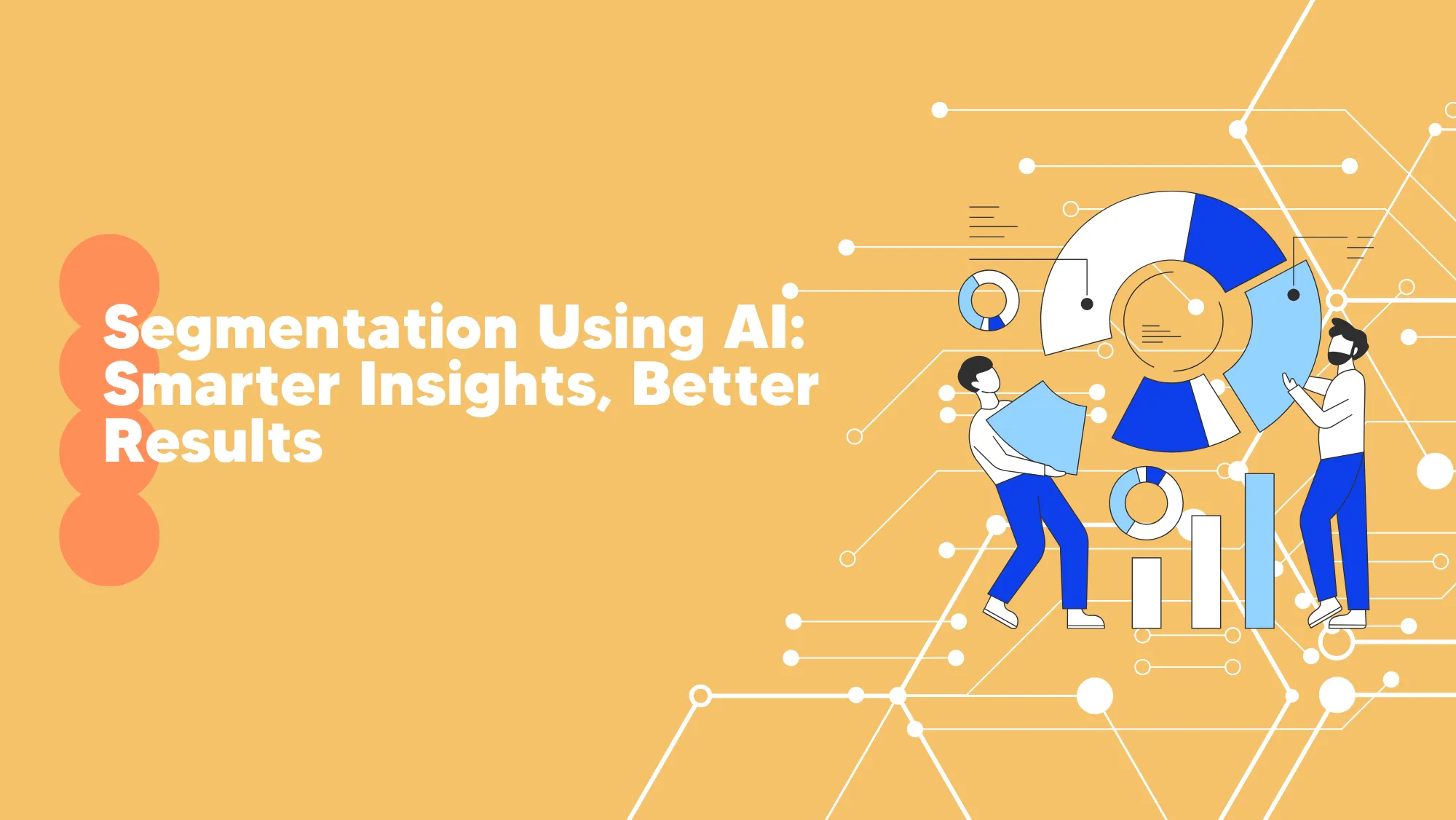
Table of Contents
Customer segmentation using AI is a popular technique for developing targeted audience groups. Companies divide their clientele into discrete groups according to preferences, demographics, or behaviors. According to research, segmented marketing can raise income by an astounding 760%, and segmentation can increase conversion rates by as much as 50%.
The article emphasizes the revolutionary role that machine learning and deep learning play in customer segmentation and delves into the workings of AI-driven consumer segmentation.
AI enables marketers to spot trends, forecast actions, and create memorable, highly customized experiences. We’ll look at how AI-powered customer segmentation operates, why it’s revolutionary, and how it’s producing better outcomes and more insightful data in today’s cutthroat market.
What is Customer Segmentation Using AI?
Artificial intelligence is used in customer segmentation, which separates a company’s clientele into distinct groups according on intricate patterns in their interactions, preferences, and behavior.
In contrast to traditional segmentation, which frequently depends on straightforward categories like age or geography, AI-driven segmentation digs deeper by examining vast volumes of data to find potentially undetected data.
Artificial intelligence can comprehend consumer behavior by utilizing algorithms that automatically identify and adapt to new patterns. Thanks to machine learning and deep learning, companies may identify patterns in large datasets without human labor. This aids in creating exact and adaptable segments that can change to meet customers’ evolving demands and interests.
Thereby, AI-driven segmentation gives companies a greater understanding of their clientele, empowering them to develop more accurate, successful, and customized marketing plans.
How Machine Learning Fuels Precise Segmentation
Machine learning (ML) plays a central role in AI-driven customer segmentation by processing vast amounts of data to uncover patterns and trends that are difficult for human analysts to detect.
Unlike traditional methods that rely on preset rules and manual categorization, machine learning uses algorithms that can sort through complex datasets to reveal meaningful insights about customer behavior, preferences, and trends.
The Operation of Machine Learning Algorithms in Segmentation
Machine learning algorithms are quite good at identifying patterns in data. Clusters can be found, behavioral changes can be detected, and connections between various client attributes can be revealed.
To help businesses comprehend different client needs, the K-means clustering algorithm, for instance, may create accurate segments by grouping customers based on similarities, including product preferences or frequency of purchases.
Source: Researchgate.net
Another helpful approach is to use decision trees to categorize customers based on different decision points, like their buying patterns, responses to promotions, or support inquiries. These models learn to identify the traits of high-value customers, those likely to leave, or those open to new product offerings without relying on predefined rules.
Source: Researchgate.net
Improving Segmentation Accuracy with Machine Learning
Machine learning enables a more accurate segmentation process by continuously learning and adapting to new data. Here are some specific ways machine learning improves segmentation:
-
Identifying Hidden Patterns
ML algorithms analyze vast amounts of customer data—everything from browsing history to purchasing habits. This allows them to detect subtle trends that human analysis might miss. For instance, ML might reveal that certain groups of customers respond well to specific marketing channels or product types.
-
Automating Segmentation
Segmentation can be automated because traditional segmentation is frequently static and needs to be updated manually regularly. In contrast, ML-based segmentation automatically adapts to new data, making companies aware of evolving consumer preferences without requiring time-consuming, periodic updates.
-
Creating Micro-Segments
With machine learning, businesses can drill down into smaller, highly specific customer groups, known as micro-segments. For instance, a micro-segment might consist of customers who purchase seasonal products only during sales events. These nuanced groups can be targeted with precise messaging, boosting engagement.
By leveraging machine learning, businesses achieve a level of segmentation detail and accuracy that enables them to create highly personalized experiences, ultimately driving better marketing results and improving customer satisfaction.
Deep Learning in Customer Segmentation
Deep learning, an advanced branch of artificial intelligence, raises the bar for customer segmentation and enables companies to establish even more precise and intricate consumer groups or micro-segments.
While basic machine learning and traditional segmentation can identify broad customer groupings, deep learning goes further, uncovering connections and patterns in data that would otherwise go unnoticed.
How Deep Learning Identifies Micro-Segments
Deep learning algorithms process unstructured data from various sources, such as social media, browsing patterns, or purchase histories. Using this data, they identify micro-segments that represent highly specific customer groups.
For instance, a deep learning model could reveal a segment of eco-conscious shoppers who respond best to email promotions but tend to purchase only during sales events.
The Benefits of Deep Learning for Hyper-Personalized Marketing
Deep learning makes hyper-personalized marketing possible by exposing such subtle micro-segments, allowing companies to customize their outreach to match the tastes of each client group precisely. Here are a few useful advantages:
-
Deeper Insights into Customer Preferences
Deep learning reveals patterns that may not be visible using simpler methods. For instance, it might identify customers who are influenced by specific trends, allowing companies to align their marketing strategies with these preferences.
-
Improved Engagement through Targeted Content
By using deep learning, marketers can create content and promotions specifically designed for each micro-segment. For example, a fitness brand might discover a customer segment that prefers workout tutorials via app notifications and tailor its approach accordingly.
-
Automatic Adaptation to New Data
Deep learning models adapt on their own as client behavior evolves. This guarantees that segmentation stays current, enabling companies to adapt to changing consumer preferences or market trends without making frequent human modifications.
Deep learning goes beyond traditional segmentation by consistently improving customer insights and providing accurate recommendations that speak to each individual. This advanced degree of segmentation ultimately improves consumer connections by facilitating more efficient, tailored interactions.
Benefits of AI-Powered Customer Segmentation
AI-driven customer segmentation brings several advantages that help businesses improve their marketing strategies:
- More Accurate Targeting: AI can analyze vast data to identify specific customer segments, ensuring that marketing efforts are more focused and relevant.
- Better Customer Engagement: With AI, businesses can tailor their interactions and offers to match customer preferences, leading to stronger relationships and increased engagement.
- Higher Conversion Rates: AI helps target high-value customers with personalized content, boosting the likelihood of converting leads into paying customers.
- Efficiency of Operations: Businesses can make more rapid, data-driven decisions by using AI to automate segmentation, which saves time and money.
- Uncover Hidden Patterns: AI can detect complex patterns and emerging trends, revealing customer segments that may have been overlooked manually.
Steps to Start with AI Customer Segmentation
-
Get your data ready
The most crucial initial step is to collect and tidy your data. Ensure that your consumer data is current and correct. To prepare the format for analysis, you might need to fill in the blanks, eliminate duplicates, and standardize it.
-
Specify Your Objectives
It’s critical to have a clear goal before implementing AI. Are you trying to understand customer preferences better, find high-value clients, or enhance focused marketing campaigns? Clearly defining goals will direct the rest of the segmentation procedure.
-
Choose the Right AI Tools
Select AI tools and platforms that best fit your needs. You may need machine learning software or platforms like Python, TensorFlow, or cloud-based AI services. Consider factors like ease of use, scalability, and integration with your existing systems.
-
Select the Segmentation Algorithm
Based on your data and goals, choose the appropriate AI algorithm. Popular choices for segmentation include clustering algorithms like K-means or Decision trees. Some AI models can identify complex patterns without requiring labeled data, while others work better with structured input.
-
Train Your Model
After selecting an algorithm, enter the data you have prepared into it. After analyzing the data, the AI model will divide the client base into various groups according to shared characteristics. Depending on how complicated the data and model are, this stage could take a while.
-
Evaluate the Results
After training the model, assess the quality of the customer segments. Check if the segments are meaningful and align with your business goals. You can use metrics like the silhouette score or visual tools to see if the clusters make sense.
-
Refine and Apply Insights
If necessary, improve your model. You might occasionally need to add fresh data or modify the algorithm to get better results. After you’re satisfied with the segmentation, begin using the data to improve customer experiences, develop focused campaigns, and personalize offerings in your marketing plans.
-
Keep an eye on and update
AI models improve over time, but they require constant observation. Review your segments’ performance regularly and make necessary updates based on new customer information or shifting market dynamics.
Following these steps, you can successfully implement AI-driven customer segmentation and leverage insights to boost your marketing effectiveness.
Conclusion on customer segmentation using AI
Customer segmentation powered by AI has the potential to revolutionize how companies view and interact with their clientele. By analyzing massive datasets with complex algorithms, AI can reveal hidden patterns, spot micro-segments, and offer previously impossible insights. This helps companies to develop individualized marketing plans, enhance client interaction, and increase conversion rates.
AI-powered segmentation is a crucial tool for maximizing marketing efforts and precisely reaching your audience. Discover now how AI may improve your consumer relationships and assist you in making more informed decisions.